Companies are approaching AI transformation with incomplete information. After extensive conversations with organizations across industries, I think four key facts explain what's really happening with AI adoption:
AI boosts work performance. How do we know? For one thing, workers certainly think it does. A representative study of knowledge workers in Denmark found that users thought that AI halved their working time for 41% of the tasks they do at work, and a more recent survey of Americans found that workers said using AI tripled their productivity (reducing 90-minute tasks to 30 minutes). Self-reporting is never completely accurate, but we have other data from controlled experiments that suggest gains among product development, sales, and consulting, as well as for coders, law students, and call center workers.
A large percentage of people are using AI at work. That Danish study from a year ago found that 65% of marketers, 64% of journalists, and 30% of lawyers, among others, had used AI at work. The study of American workers found over 30% had used AI at work in December, 2024, a number which grew to 40% in April, 2025. And, of course, this may be an undercount in a world where ChatGPT is the fourth most visited website on the planet.
There are more transformational gains available with today’s AI systems than most currently realize. Deep research reports do many hours of analytical work in a few minutes (and I have been told by many researchers that checking these reports is much faster than writing them); agents are just starting to appear that can do real work; and increasingly smart systems can produce really high-quality outcomes.
These gains are not being captured by companies. Companies are typically reporting small to moderate gains from AI so far, and there is no major impact on wages or hours worked as of the end of 2024.
How do we reconcile the first three points with the final one? The answer is that AI use that boosts individual performance does not naturally translate to improving organizational performance. To get organizational gains requires organizational innovation, rethinking incentives, processes, and even the nature of work. But the muscles for organizational innovation inside companies have atrophied. For decades, companies have outsourced this to consultants or enterprise software vendors who develop generalized approaches that address the issues of many companies at once. That won’t work here, at least for a while. Nobody has special information about how to best use AI at your company, or a playbook for how to integrate it into your organization. Even the major AI companies release models without knowing how they can be best used. They especially don’t know your industry, organization, or context.
We are all figuring this out together. So, if you want to gain an advantage, you are going to have to figure it out faster than everyone else. And to do that, you will need to harness the efforts of Leadership, Lab, and Crowd - the three keys to AI transformation.
Leadership
Ultimately, AI starts as a leadership problem, where leaders recognize that AI presents urgent challenges and opportunities. One big change since I wrote about this topic months ago is that more leaders are starting to recognize the need to address AI. You can see this in two viral memos, from the CEO of Shopify and the CEO of Duolingo, establishing the importance of AI to their company’s future.
But urgency alone isn't enough. These messages do a good job signaling the 'why now' but stop short of painting that crucial, vivid picture: what does the AI-powered future actually look and feel like for your organization? My colleague Andrew Carton has shown that workers are not motivated to change by leadership statements about performance gains or bottom lines, they want clear and vivid images of what the future actually looks like: What will work be like in the future? Will efficiency gains be translated into layoffs or will they be used to grow the organization? How will workers be rewarded (or punished) for how they use AI? You don’t have to know the answer with certainty, but you should have a goal that you are working towards that you are willing to share. Workers are waiting for guidance, and the nature of that guidance will impact how The Crowd adopts and uses AI.
An overall vision is not enough, however, because leaders need to start to anticipate how work will change in a world of AI. While AI is not currently a replacement for most human jobs, it does replace specific tasks within those jobs. I have spoken to numerous legal professionals who see the current state of Deep Research tools as good enough to handle portions of once-expensive research tasks. Vibe coding changes how programmers allocate time and effort. And it is hard to not see changes to marketing and media work in the rapid gains in AI video. For example, Google’s new Veo 3 created this short video snippet, sound and all, from the text prompt: An advertisement for Cheesey Otters, a new snack made out of otter shaped crackers. The commercial shows a kid eating them, and the mom holds up the package and says "otterly great"
Yet the ability to make a short video clip, or code faster, or get research on demand, does not equal performance gains. To do that will require decisions about where Leadership and The Lab should work together to build and test new workflows that integrate AIs and humans. It also means fundamentally rethinking why you are doing particular tasks. Companies used to pay tens of thousands of dollars for a single research report, now they can generate hundreds of those for free. What does that allow your analysts and managers to do? If hundreds of reports aren’t useful, then what was the point of research reports?
I am increasingly seeing organizations start to experiment with radical new approaches to work in response to AI. For example, dispersing software engineering teams, removing them from a central IT function and instead having them work in cross-functional teams with subject matter experts and marketing experts. Together, these groups can “vibework” and independently build projects in days that would have taken months of coordination across departments. And this is just one possible future for work. Leaders need to describe the future they want, but they also don’t have to generate every idea for innovation on their own. Instead, they can turn to The Crowd and The Lab.
The Crowd
Both innovation and performance improvements happen in The Crowd, the employees who figure out how to use AI to help get their own work done. As there is no instruction manual for AI (seriously, everyone is figuring this out together), learning to use AI well is a process of discovery that benefits experienced workers. People with a strong understanding of their job can easily assess when an AI is useful for their work through trial and error, in the way that outsiders (and even AI-savvy junior workers) cannot. Experienced AI users can then share their workflows and AI use in ways that benefit everyone.
Enticed by this vision, companies (including those in highly regulated industries1) have increasingly been giving employees direct access to AI chatbots, and some basic training, in hopes of seeing The Crowd innovate. Most run into the same problem, finding that the use of official AI chatbots maxes out at 20% or so of workers, and that reported productivity gains are small. Yet over 40% of workers admit using AI at work, and they are privately reporting large performance gains. This discrepancy points to two critical dynamics: many workers are hiding their AI use, often for good reason, while others remain unsure how to effectively apply AI to their tasks, despite initial training.
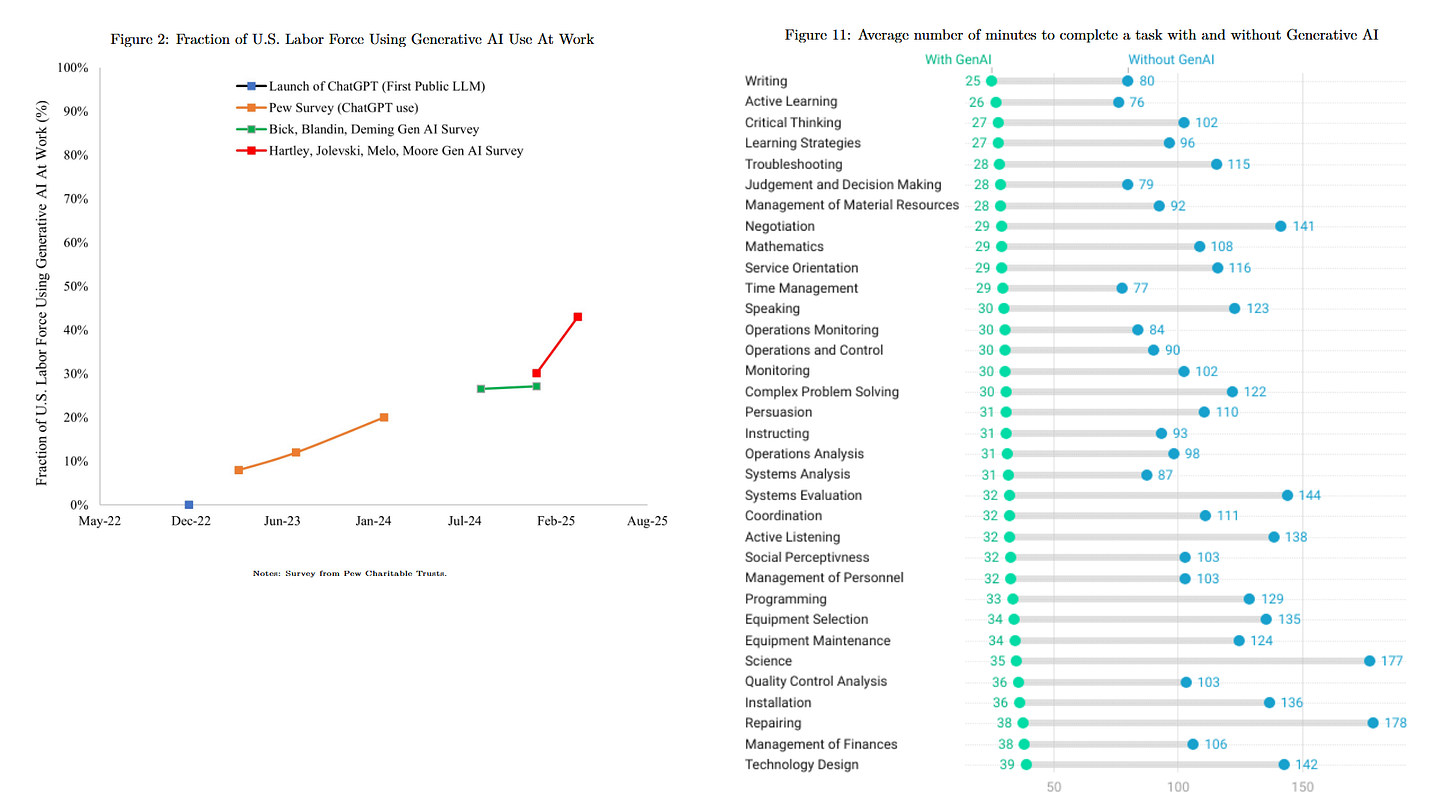
These are problems that can be solved by Leadership and the Lab.
Solving the problem of hidden AI use (what I call “Secret Cyborgs”) is a Leadership problem. Consider the incentives of the average worker. They may have received a scary talk about how improper AI use might be punished, and they don’t want to take any risks. Or maybe they are being treated as heroes at work for their incredible AI-assisted outputs, but they suspect if they tell anyone it is AI, managers will stop respecting them. Or maybe they know that companies see productivity gains as an opportunity for cost cutting and suspect that they (or their colleagues) will be fired if the company realizes that AI does some of their job. Or maybe they suspect that if they reveal their AI use, even if they aren’t punished, they won’t be rewarded. Or maybe they know that even if companies don’t cut costs and reward their use, any productivity gains will just become an expectation that more work will get done. There are more reasons for workers to not use AI publicly than to use it.
Leadership can help. Instead of vague talks on AI ethics or terrifying blanket policies, provide clear areas where experimentation of any kind is permitted and be biased towards allowing people to use AI where it is ethically and legally possible. Leaders also should consider training less an opportunity to learn prompting techniques (which are valuable but getting less important as models get better at figuring out intent), but as a chance to give people hands-on AI experience and practice communicating their needs to AI. And, of course, you will need to figure out how you will reassure your workers that revealing their productivity gains will not lead to layoffs, because it is often a bad idea to use technological gains to fire workers at a moment of massive change. Build incentives, even massive incentives (I have seen companies offer vacations, promotions, and large cash rewards), for employees who discover transformational opportunities for AI use. Leaders can also model use themselves, actively using AI at every meeting and talking about how it helps them.
Even with proper vision and incentives, there will still be a substantial number of workers who aren’t inclined to explore AI and just want clear use cases and products. That is where The Lab comes in.
The Lab
As important as decentralized innovation is, there is also a role for a more centralized effort to figure out how to use AI in your organization. Unlike a lot of research organizations, The Lab is ambidextrous, engaging in both exploration for the future (which in AI may just be months away) and exploitation, releasing a steady stream of new products and methods. Thus, The Lab needs to consist of subject matter experts and a mix of technologists and non-technologists. Fortunately, the Crowd provides the researchers, as those enthusiasts who figure out how to use AI and proudly share it with the company are often perfect members of The Lab. Their job will be completely, or mostly, about AI. You need them to focus on building, not analysis or abstract strategy. Here is what they will build:
Take prompts and solutions from The Crowd and distribute them widely, very quickly. The Crowd will discover use cases and problems that can be turned into immediate opportunities. Build fast and dirty products with cross-functional teams, centered around simple prompts and agents. Iterate and test them. Then release them into your organization and measure what happens. Keep doing this.
Build AI benchmarks for your organization. Almost all the official benchmarks for AI are flawed, or focus on tests of trivia, math or coding. These don’t tell you which AI does the best writing or can best analyze a financial model or can help guide a customer making purchases. You need to develop your own benchmarks: how good are each of the models at the tasks you actually do inside of your company? How fast is the gap closing? Leadership should help provide some guidance, but ultimately The Lab will need to decide what to measure and how. Some benchmarks will be objective (Anthropic has a guide to benchmarking that can help as a starting place), but it is also fine for some complex benchmarks to be “vibes alone,” based on experience.
For example, I “vibe benchmarked” Manus, an AI agent based on Claude, on its ability to analyze new startups by giving it a hard assignment and evaluating the results. I gave it a short description of a fictional startup and a detailed set of projected financials in an Excel file. These materials came from a complex business simulation we built at Wharton (and never shared online) that took teams of students dozens of hours to complete. I was curious if the AI could figure it out. As guidance, I gave it a checklist of business model elements to analyze, and nothing else.
In just a couple of prompts, Manus developed a website, a PowerPoint pitch deck, an analysis of the business model, and a test of the financial assumptions based on market research. You can see it at work here. In my evaluations of the work, the 45 page business model analysis was very solid. It was not completely free from mistakes, but has far less mistakes, and is far more thorough, than what I would expect from talented students. I also got an initial draft website, the requested PowerPoint, and a Deep Dive in financial assumptions. Looking through these helped me find weak spots — image generation, a tendency to extrapolate answers without asking me — and strong ones. Now, every time a new agentic system comes out, I can compare it to Manus and see where things are heading.
Go beyond benchmarks to build stuff that doesn’t work… yet. What would it look like if you used AI agents to do all the work for key business processes? Build it and see where it fails. Then, when a new model comes out, plug it into what you built and see if it is any better. If the rate of advancement continues, this gives you the opportunity to get a first glance at where things are heading, and to actually have a deployable prototype at the first moment AI models improve past critical thresholds.
Build provocations. Many people haven't truly engaged with AI's potential. Demos and visceral experiences that jolt people into understanding how AI could transform your organization, or even make them a little uncomfortable, have immense value in sparking curiosity and overcoming inertia. Show what seems impossible today but might be commonplace tomorrow.
Re-examining the organization
The truth is that even this framework might not be enough. Our organizations, from their structures to their processes to their goals, were all built around human intelligence because that's all we had. AI alters this fundamental fact, we can now get intelligence, of a sort, on demand, which requires us to think more deeply about the nature of work. When research that once took weeks now takes minutes, the bottleneck isn't the research anymore, it's figuring out what research to do. When code can be written quickly, the limitation isn't programming speed, it's understanding what to build. When content can be generated instantly, the constraint isn't production, it's knowing what will actually matter to people.
And the pace of change isn't slowing. Every few months (weeks? days?) we see new capabilities that force us to rethink what's possible. The models are getting better at complex reasoning, at working with data, at understanding context. They're starting to be able to plan and act on their own. Each advance means organizations need to adapt faster, experiment more, and think bigger about what AI means for their future. The challenge isn't implementing AI as much as it is transforming how work gets done. And that transformation needs to happen while the technology itself keeps evolving.
The key is treating AI adoption as an organizational learning challenge, not merely a technical one. Successful companies are building feedback loops between Leadership, Lab, and Crowd that let them learn faster than their competitors. They are rethinking fundamental assumptions about how work gets done. And, critically, they're not outsourcing or ignoring this challenge.
The time to begin isn't when everything becomes clear - it's now, while everything is still messy and uncertain. The advantage goes to those willing to learn fastest.
When I talk to companies, the General Counsel's office is often the choke point that determines AI success. Many firms still ban AI use for outdated privacy reasons (no major model trains on enterprise or API data, and you can get fully HIPAA etc. compliant versions). While no cloud software is without risk, there are risks in not acting: shadow AI use is nearly universal, and all of the experimentation and learning is kept secret when the company doesn’t allow AI use. Fortunately, there are lots of role models to follow, including companies in heavily regulated industries that are adopting AI across all functions of their firm.
Your Leadership-Lab-Crowd triangle perfectly describes the gap I'm seeing on Wall Street. Only some large banks and PE funds have rolled out internal chatbots or parter with firms creating financial ai tools - but even there, uptake is tiny because the way they're presented to employees is as "optional sidekicks". When the message is “play with it on your own time,” no one pulling 80-hour weeks willingly does so.
The deeper blocker is psychological. Junior staff worry that using AI to do grunt work will short-circuit the skills they’re supposed to master. However, not all grunt work is of equal value when it comes to skillbuilding. Moreover, the skill that will matter most in the years coming will be knowing how to direct, audit, and iterate AI outputs. Leadership has to make that explicit - shift the truly mind-numbing grunt work to AI, keep the judgment-building parts in human hands, and treat “managing the machine” as the new apprenticeship. And that kind of sorting process won’t happen if the message from the top is “try using AI if you want, when you want”. It needs an org-wide mandate and protected forums: AI discussion committees & innovation sessions, where teams test, map, and share what works regularly
I make the same case in my substack post "Grunt Work & Growth" and would love your take if you have a minute. Thanks for pushing the conversation forward!
Great article. We are in the "faster horses" phase of Ai, in reference to Henry Ford's comment, "If I asked my customers what they wanted, they would say a faster horse."
Everyone is just imagining how Ai will make their old work faster, not really understanding the impact of the changes. If you use Ai to fill out a document, and the other person uses Ai to read the document, then why do we need the document at all? Some documents will still be needed of course, but maybe not the ones that could be easily automated.
This is the time to rethink the entire process. There is still too much emphasis on reverse engineering Ai to do pointless work faster and in higher volume.
Looking back to previous technological automations, think of the intense precision and careful thought required to get an automated packaging line to operate properly. The WORK is now in the design of the task, not in the doing of the task.
The best humans could never match the output of an automated filling line. But a poorly thought out design can lead to choke points and piles of broken bottles that takes longer to fix, and is more expensive, than just filling the bottles by hand.
Doing bad or pointless work faster or more frequently is not the goal.