Thinking companion, companion for thinking
Some simple ways to use AI to break you out of biases
I am sure most of my readers are aware of cognitive biases, areas where human decision-making veers from the rational. There are ongoing arguments on how many fundamental biases there are (150? 25? Just 1?), but there is no doubt that humans often fail to behave rationally. And, as many of my colleagues have documented, these failures can lead to bad decision-making. So much so that trying to reduce cognitive biases is a major theme in many fields of social science.
I would like to suggest that AI provides a unique opportunity to address the issues of decision biases in a new way. After all, many of these biases come from us being stuck in our own mind. But now we have another (strange, artificial) mind we can turn to for help. AI can assist us as a thinking companion to improve our own decision-making, helping us reflect on our own choices (rather than simply relying on the AI to make choices for us). In this post, I’ll discuss a couple of approaches that might be useful, but it is just a start. We are suddenly in an area where human decision-making skills can be easily augmented in a new way, and the potential, and risks, are not completely clear.
AI for Challenging our Thinking
There are many different reasons why we tend to prefer information that does not challenge our assumptions too much, from confirmation bias to the IKEA Effect (we think anything we make or build is of higher quality) to the Fundamental Attribution Error (we attribute our failures to luck, and the failures of others to skill.) As a result, we don’t pay enough attention to the fact that we may be wrong, or explore alternatives, which can lead to a failure to plan, or assess our chances realistically.
These sorts of biases can be even stronger on teams, where the Abilene Paradox and Groupthink both impact the willingness of teams to offer alternatives. The Abilene Paradox is a phenomenon in which a group of people collectively decide on a course of action that is counter to the preferences of any individual member of the group. This occurs because each member mistakenly believes that their own preferences don’t fit the group consensus and therefore, they do not want to voice their opposition. So everyone decides on an action that each individual group member hates! Groupthink, on the other hand, happens when the desire for harmony or conformity leads to irrational or dysfunctional decision-making. Members of the group prioritize agreement over critical evaluation of alternative viewpoints, often suppressing dissenting opinions and overlooking potential problems.
These biases share a common thread of favoring information that aligns with preconceived notions, suppressing dissenting opinions, and limiting the exploration of diverse perspectives. We know of some techniques that can help break these biases, but they are not easy to do because they require acknowledging the problem in the first case. For example, conducting a pre-mortem, where you tell stories about how a project might fail, is linked to higher success rates, but requires acknowledging failure. This is precisely the value of the exercise, however, because it makes it okay to consider possible negative outcomes, breaking through your biases.
AI is very good at giving you stories of failure (and possible solutions). For example: I am going to write a blog post on how to use AI to make better decisions that will go out to 30,000 people. Give me four vivid stories of how writing this blog post might fail badly, either in process or in outcome.
I found the answers plausible enough that I asked AI for help in solving them. I pasted in the section above, and asked it: Here is what I am planning on writing, am I still at risk for these failures? Offer specific language I should add or change.
There are other prompts that you can use to have AI challenge your thinking. Paste in a project plan and write: give me three paragraphs, each of which explains in detail one distinct way in which this project failed, focusing on failures of [process/sales/planning/etc]. Give it your resume and ask what skill gaps do I have that might stop you from achieving my goal of ____ . Bring a laptop into your next sprint review and give it the transcript of your meeting and ask what risks are we missing in this transcript of achieving our goals of _____.
AI for Embracing Change
On the flip side, there are also many biases that suggest that worrying too much about failure can paralyze us from taking action. Among these biases are loss aversion (our tendency to avoid losses beats our willingness to seek gains), hyperbolic discounting (our tendency to consider short-term awards more than long-term benefits), and the status-quo bias that causes us to avoid making changes, even when they might be good.
These biases can cause us to overlook opportunities, but AI can help. I have recently written about the practical ways that AI can help get us unstuck and advance our goals. But AI can also be useful in directly overcoming biases that prevent us from embracing change.
For example, we can ask it to help us beat status quo bias by framing our failure to act as a loss, a frame which also helps us overcome loss aversion by considering gains. AI is surprisingly good at this: I was thinking of starting to train for a 5k, but I am very busy and don't think I want to make such a large change. Can you reframe my failure to engage in a 5k as a loss, rather than a default option? Make the framing vivid.
If you put it that way…
AI for Considering Alternatives
There are also biases that limit our view of alternative options, and blind us to the true costs of our actions. It is no wonder that this paper surveyed every economist in Sweden to find the most important economic concept for everyone to learn discovered the overwhelming choice was... opportunity cost.
Opportunity cost is what you give up when you make a choice. Not just what you didn't buy with the money you spent, but also what you could have done with that money if you saved it for the future. Since, in a rational world, you must have chosen the best option, opportunity cost is your next best choice. Except, of course, that isn’t really true in actual human decisions. A lot of research has shown that people neglect opportunity cost when making choices. This leads to consumers falling prey to bad marketing, policy makers selecting short-sighted decisions, and other bad consequences.
The concept of opportunity cost is notoriously difficult to use in practice, since it requires actively considering uncomfortable and annoying information that undermine the joy of acting on our choices. For example, to consider opportunity costs, you could list other alternatives you would want to spend money on, put costs in terms of hours of work, or even imagine yourself when retired. Not only are these tedious to consider, but they can be tedious to calculate. Again, this is where AI comes in. You can ask the AI: I am 25 and making $22 an hour. I want to buy a new gaming PC for $2,800. How could I think of the opportunity cost of making the purchase both in terms of my work now, and in terms of my eventual retirement. Make whatever assumptions you need.
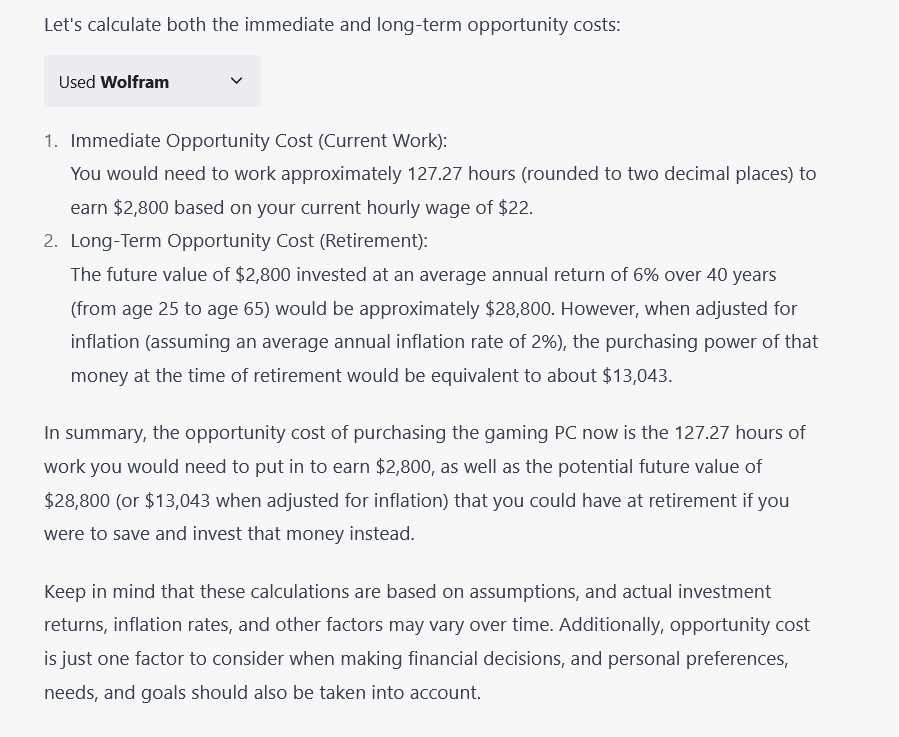
Given that the process of considering opportunity cost is designed to rob the joy out of purchases, it is not surprising that asking an AI to consider these issues is not fun. But research suggests that considering opportunity costs leads to better decisions, and it is very hard to communicate these topics in other ways (trust me, I have been involved in multiple efforts to build games to teach financial literacy).
An Augmented Conclusion
While AI can significantly enhance our decision-making processes by providing objective analysis and recommendations, it's important to remember that it is a tool to support human decision-makers, not replace them. AI has its limitations, and its effectiveness depends on the quality of data and the design of algorithms. Further, as we explore the potential of AI in decision-making, it's crucial to consider the ethical implications, such as algorithmic biases, data privacy, and the impact on jobs. Most importantly, AI is not meant to replace human expertise but rather to augment it. By leveraging AI's analytical capabilities, decision-makers can make more informed choices while still relying on their intuition, experience, and judgment to navigate complex situations.
If you have read the early portion of the post, you will note that the above paragraph is, verbatim, the text that the AI advised me to write to avoid the risks of failure discussed earlier. I don’t disagree with its sentiments, however, but I think it is worth concluding with a final (human) thought. We need to embrace and explore the ways in which AI can augment our decisions, while being careful of the risks, both obvious and non-obvious. People have flaws in decision-making, but so do AIs. We can hope that our flaws counterbalance each other, leaving humans as better decision-makers, without compromising our ability to control our own choices. Using AI to help us reflect on our own decision-making process seems like the safest, and most useful, way to start.
A complementrary strategy I figured out yesterday is to preface your prompt with the instruction that ChatGPT ought to ask you clarifying questions before it generates its output. Below is an example of this strategy. (I tried to format this as clearly as possible in spite of Substack's commenting limits; let me know if anything is unclear, and I will try to clarify in further comments.)
Example: "I have a complicated project for you. I'm going to provide you the instructions, but before you act upon them, I want you to ask me some clarifying questions. Do you understand this?"
Its response: "Yes, I understand. Please provide the instructions for your complicated project, and I will ask you clarifying questions to ensure I fully understand the task before taking any action."
Prompt: I want you to provide an analysis of how New York City's municipal finances work. Focus especially on the revenue side, i.e., taxes. The question at hand is this: given the rise of remote work, and the concomitant decline in office use, will taxes from offices and commercial real estate decline? And, if those taxes do decline, how will New York City make up the shortfall? Use NYC's 1970s municipal finance crisis as a comparison for your analysis.
Its clarifying questions:
Thank you for providing the details of your project. To ensure I fully understand the scope and requirements, I have a few clarifying questions:
1. Would you like me to focus on specific types of taxes, such as property taxes or income taxes, or should I provide a broader overview of all taxes related to offices and commercial real estate in New York City?
2. Should I consider any specific geographic areas within New York City or analyze the city as a whole?
3. Are there any timeframes or specific years you would like me to use for comparison, or should I just focus on the general trends of remote work and its impact on office use?
4. In terms of the 1970s municipal finance crisis, would you like a brief overview of the crisis itself, or just a comparison between the potential decline in taxes from offices and commercial real estate and the financial challenges faced during that time?
5. Do you have any preferences for the format of the analysis, such as a structured report, a list of key points, or a more conversational approach?
Please let me know if there's any additional information or context you'd like me to consider in my analysis.
Nice write-up! I can envision a browser plugin that review sentences I type and offer me suggestions when I pause to think.
For example, if I am making a logic mistake, it suggests a better version. If I am rambling, it proposes a simpler version that is easier to read (playing the role of my audience.)
Maybe it's time to start an experiment on github. :-)